The Rising Cost of GPU Real Estate
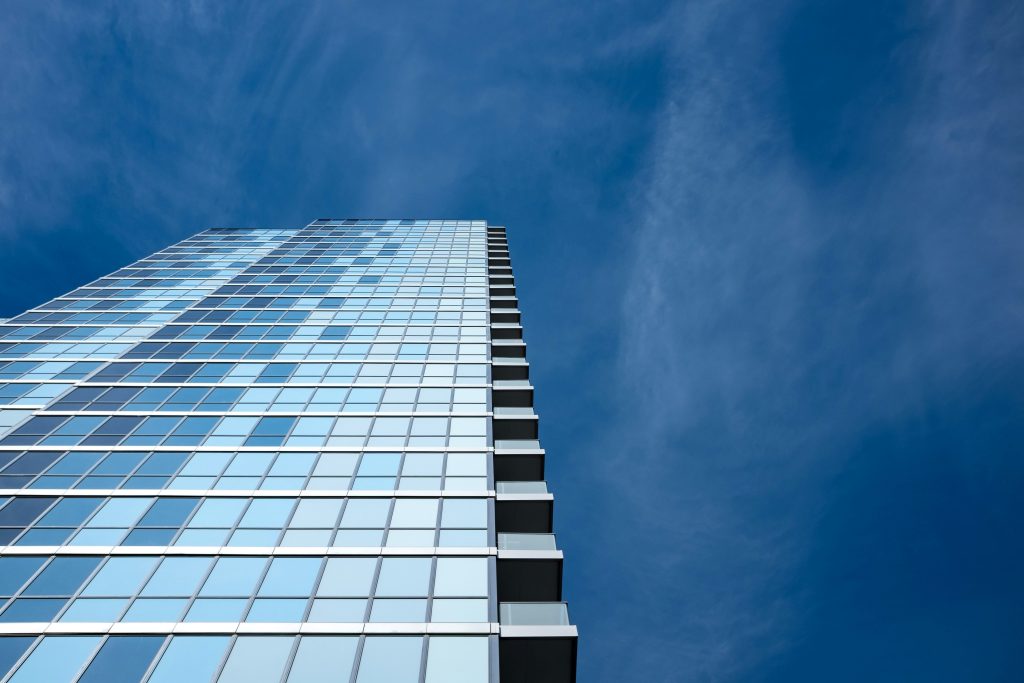
The demand for accelerators such as Graphics Processor Units (GPUs) has never been higher and continues to grow. This demand is outpacing the available manufacturing capacity of the foundries that are producing the silicon used in GPUs. When the demand for anything outpaces the supply, the knock-on effect is an inevitable increase in price.
This huge GPU demand, predominantly by a very small number of consumers of a very large number of GPUs, is globally driving up the price of accelerators, in particular, GPUs. A growing number of large cloud providers are also now consuming more and more GPUs as they begin to provide services for AI, ML, LLM and other computationally intensive workloads. Pricing of higher-end GPUs has continued to increase, with secondary markets asking for multiples of the original price of the GPU. So, if you have GPUs, you want to put them into systems that get the maximum value out of that GPU.
GPUs represent a significant cost in the capital expenditure and the operational cost of data centers and data center management. Maximizing this investment over time is imperative, and the conventional approach to data center infrastructure struggles in this regard. A disaggregated approach to GPU management, such as Cerio’s CDI Platform solution, has been designed with this exact purpose in mind: maximize the ROI of the GPU investment within the data center.
At the same time, there is an arms race going on between the traditional CPU vendors. They’re racing against each other to provide higher capacity, power, frequency, and more complex CPUs which is driving up the cost of servers put in these expensive GPUs.
The cost of operating GPUs is steadily climbing, driven by multiple factors. One key issue is that silicon-based compute systems are fundamentally designed around the power and cooling capacity they can support. As a result, the expenses associated with these systems—along with the power and cooling infrastructure they require—continue to increase. Additionally, the growing demand for robust infrastructure to house the servers running these GPUs further contributes to escalating costs.
For example, the cost of a server may only represent a quarter of the total cost of ownership of that server in the data center. One must consider all the other elements that contribute to the server’s total cost of ownership: power and cooling, facilities, support and software costs, for example. These factors triple the original purchase price of the server when looking at the total cost of ownership. If you look at it from the perspective of how many GPUs you can get into a server (with the max being 8), that server is a very expensive place to put those GPUs. Not only is it expensive in terms of the acquisition cost of the technology, products, and infrastructure to support it, but also the operational cost of having all those really expensive GPUs in one system.
When failures occur, such as a GPU malfunction or the breakdown of another critical component in the server—be it a memory card, storage device, or another essential part—the impact is significant. The entire server, including all its GPUs, is taken offline until the repair process is completed, leading to costly operational downtime, potential disruptions of workloads and customer dissatisfaction.
The demand for GPUs is rapidly increasing, both in terms of application workloads and the amount of time these systems remain powered on. As a result, we’re observing a reduction in Mean Time Between Failures (MTBF) in these high-cost GPU systems, which significantly adds to the indirect costs of running GPU servers.
When combined with the rising expense of infrastructure, the escalating costs of GPUs themselves, and the heightened downtime risks in densely packed systems, the overall cost of operating GPU servers is growing at an alarming rate. This trend highlights that packing more GPUs into a single system greatly amplifies these cost-driving factors.
Today, we face a challenge: enabling applications to access the maximum number of GPUs while ensuring the right GPUs are being used. However, this introduces another challenge—not all GPUs are created equal. Some GPUs are better suited for demanding tasks like advanced ML, AI, and analytics. Some GPUs are much more focused towards graphics and the types of applications for media and entertainment, and there’s no one system designed for both types of GPUs and workloads making it essential to match the right GPU to the right application for optimal performance. We tend to make compromises, and those compromises tend to be more expensive over time. So, the problem is that we don’t have a model that really allows us to control the cost of the server and the GPU in terms of both the number or type of GPUs, and the type of system that we need to drive those GPUs.
The next GPU cycle
Another significant cost factor lies in keeping up with the rapidly evolving world of accelerators and GPUs. GPU manufacturers introduce new technologies at nearly twice the pace of advancements in traditional server systems. Once GPUs are installed in a server, however, that server’s real estate becomes effectively locked. The expense of unracking and replacing GPUs within the server is time consuming and comes at a cost, creating a challenge for organizations trying to stay agile in adopting the latest GPU advancements.
What ends up happening is that we quickly age out equipment integrated with older GPUs, and we put new infrastructure in place to accommodate new GPUs. That’s a waste because the applications, CPUs and memory that were in those original systems may be adequate for driving these new GPUs. We find ourselves caught in an ongoing refresh cycle, either constrained by the slower pace of server upgrades or driven by the high cost of GPUs. This often forces us to refresh significant portions of our data centers at substantial expense—an investment that may not always be necessary.
How did we get here?
It’s worth noting that the system model used for servers and the GPUs integrated into them has remained largely unchanged for over 20 years.
The way that we contemplated building a server in the first place wasn’t really to support GPUs. Initially when we put two socket systems into servers, it was to provide more memory bandwidth, and that’s allowed us to have larger footprints of memory, increased the number of cores, and the speed of those cores in a server.
But that cost keeps going up relative to the performance of those servers overall. That means that everything attributed to a server, the cost of that real estate goes up because the rack space is more expensive because we may be occupying more rack space.
The number of systems you can get into a data center rack has been reduced. But the amount of power to that rack is finite, which reduces the number of systems that can be integrated into a single rack. All these challenges are diminishing the flexibility in how data centers are designed and the types of systems that can be built. Ideally, you don’t want to deploy different systems for every application—you want to move toward standardization to simplify operations and improve efficiency.
This means you’re forced to choose a design point for systems that broadly meets your needs. However, for many applications, this often results in over-provisioning infrastructure—spending more on the data center than is necessary today or even in the foreseeable future. These challenges stem from the inherent physical limitations of GPU servers, compounded by the operational complexities they introduce.
Solution
There was a time when data center design focused on concentrating technology for optimal performance, with components kept close together. However, over time, we recognized the need to distribute and disaggregate these components for greater flexibility and efficiency.
For example, when we needed to innovate in storage, we developed a new type of fabric that enabled the creation of storage area networks, allowing us to disaggregate storage from servers. This approach made it possible to logically reconnect storage to a server, making it appear as if the storage were locally within the server, even though the operating system didn’t recognize the difference. This was a game-changer for scaling storage. In fact, much of the storage infrastructure we use in data centers today still follows that model.
However, we haven’t been able to do the same with other resources inside the server, such as GPUs. Currently, there are physical limitations on how many GPUs can be installed in a server, due to constraints related to power, cooling, and the overall infrastructure required to support them. But what if we could disaggregate the GPUs from the server, reattaching them in real-time so that the operating system, applications, drivers, and BIOS would still perceive the GPUs as locally available?
This would allow us to separate the challenges of power and cooling inside the server, the physical limitations on the number of GPUs, and the difficulty of mixing different GPU types in a single system—issues that are hard to solve today. By introducing a transparent fabric, we could reattach GPUs dynamically, enabling systems to perform at the same level as if they were physically inside the server. With the right performance and agility, we could overcome many of the existing limitations in GPU deployment, ultimately altering the cost-benefit ratio of building large GPU systems.
This issue has been a major barrier for scaling GPU deployments across various industries, from cloud providers and enterprises to telecommunications and media. The constraints on GPU systems have limited the types of GPU infrastructure that can be built. But by separating GPUs from servers, we can eliminate these limitations. This decoupling would allow us to upgrade or expand GPU fleets independently from the pace at which server upgrades occur, solving most of the cost-related problems in building large-scale GPU systems.
Cerio
So, what would this kind of fabric require? At Cerio, we’ve been focused on revolutionizing the way systems and components are connected in a data center. We’ve developed a fabric with extremely low and predictable latency, along with the ability to scale through a distributed fabric. This approach reduces the complexity of building a data center class fabric that can carry traffic between systems and GPUs.
Cerio’s fabric is designed to address this specific problem—enabling the disaggregation of GPUs from servers. Unlike expanding the PCI infrastructure (which has scale limitations, high costs, and fragility), Cerio’s fabric offers true agility. It allows resources to be spread across the data center and recomposed in real-time to meet the needs of any system connected to the fabric.
With Cerio’s fabric, GPUs can be dynamically allocated based on demand, whether for long-term or time-based workloads. This allows for a more sophisticated system at a fraction of the cost, without the dependencies on large GPU systems or specific configurations. It also enables improved GPU utilization, reducing the overall cost. From an operational standpoint, the ability to easily recompose GPUs into different systems or access other GPUs if one fails eliminates the risk of major disruptions, significantly improving the reliability of the data center.
This is what Cerio built over the past 20 months. Cerio’s CDI Platform is released, and customers are now deploying our solution to leverage all the advantages of GPU disaggregation with a fabric that drives efficiency, flexibility, agility, operational efficiencies and tremendous cost savings in GPU-based systems.