Recalculating Power and Cooling in the AI Era
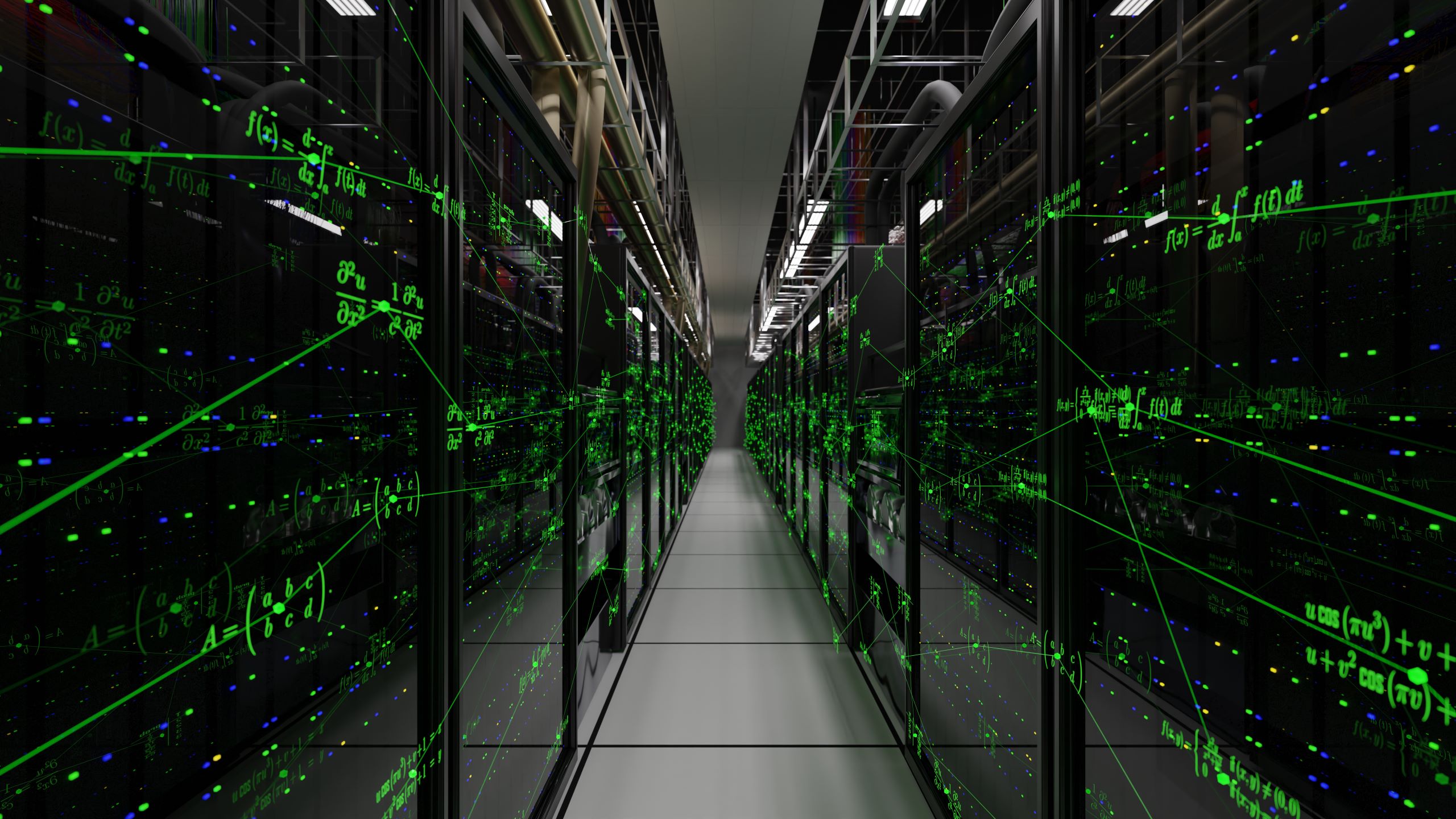
Data centers are already big consumers of energy, but that demand is expected to surge 160% by 2030, driven by accelerated computing and AI. This rising tidal wave of energy demand is fueling investments in power and cooling infrastructure that is inefficient and over-provisioned because of the fixed design of today’s data center systems. Calculating the cost of waste is increasingly critical for sustainable innovation in the AI era, forcing changes in the way data centers think about their valuable resources.
It’s time for an advanced system architecture that creates an “effective usage” model for dynamic AI infrastructure while minimizing waste in the power and cooling supply chain.
Managing capacity and consumption
In contemplating the power requirements for a data center, there are a few things to keep in mind.
Every device in the data center will consume less power when it is “idle,” more power when it is under load and significantly more when it is under peak load. However, we cannot use those numbers to plan power usage in the data center. Typically, data center operators will use the Power Supply Unit (PSU) capacity as the worst case to ensure power is available when needed. While often a judgment call, it is important to consider the power consumption of any upgrades and adjust accordingly.
There are two calculations to take into consideration, capacity and consumption.
- Capacity is the maximum possible power draw of every device in the data center. This capacity number is used to size the entire power and cooling supply chain from the utility to the device consuming the power. Capacity is what drives the infrastructure required for the data center.
- Consumption is the measurement of the actual power draw of every device in the data center, including all of the necessary conversion losses along that same supply chain. This number is what primarily drives the utility bill.
In order to provide maximum uptime for the business, the entire power supply chain must be built in a redundant manner. Most devices in the data center will have redundant power supplies that are sized so that a single power supply can handle the peak load. Each of those power supplies will be fed power from independent Rack Power Distribution Units (PDUs) and must be sized to handle the entire load of the devices plugged into it. Each of those Rack PDUs will typically be plugged into independent floor mounted “row” PDUs, each of those are often fed from two different utilities. All of this, as well as backup power (batteries, generators, flywheels or a combination), must be capable of supporting the entire power load of the data center, so if the IT equipment requires one (1) megawatt of power, then to enable redundancy you will need to have infrastructure and utility feeds of two (2) megawatts. Well, not exactly…
Data Center operators must not only worry about how much power the IT devices require, they must also worry about the “power to power” those devices and the “power to cool” those devices.
- Power to Power: At multiple points along the power supply chain, loss occurs from each power conversion (utility power to 3-phase, AC to DC, etc.) and this power loss is referred to as the “power to power” the IT equipment.
- Power to Cool: When a server consumes power to do its work, heat is generated that must be removed to keep the server operating at a temperature that keeps the components in the server healthy. The cool air goes into the front of the server, across the hot components absorbing the heat and then exhausts hot air out the back of the server. This is why rows of racks are oriented back-to-back (forming a hot aisle) and front-to-front (forming a cold aisle). The hot air from the back of the server flows into the hot aisle and is then drawn into the return plenum to the chiller where the air is cooled and then sent into the cold aisle. The cooling infrastructure also consumes power and that is referred to as “power to cool.”
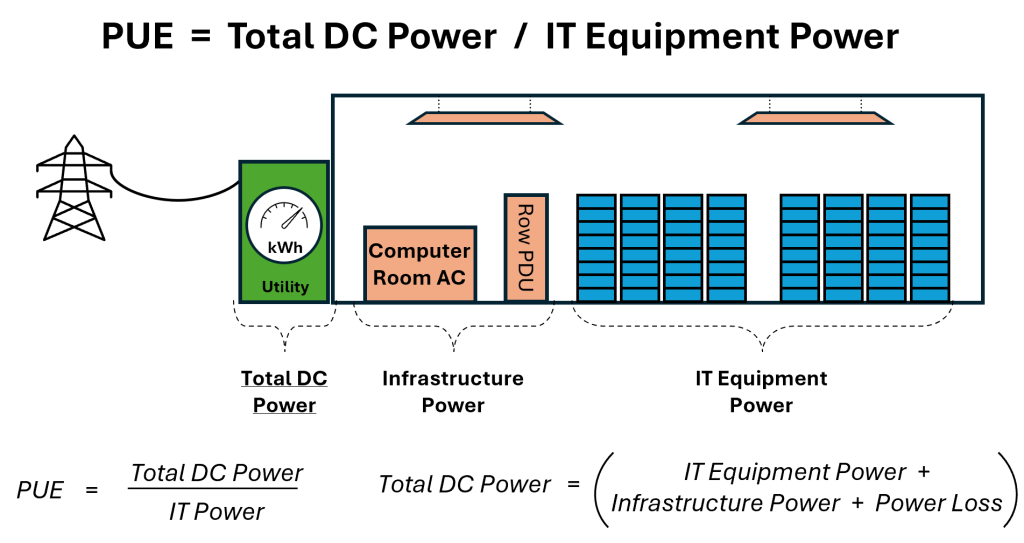
In a typical data center, for every watt of power consumed by IT devices, another watt of power is consumed by the infrastructure overhead associated with the “power to power” and the “power to cool” those IT devices. Data centers over the last decade have been very focused on reducing this overhead and measure it using Power Utilization Efficiency (PUE). While some hyperscale data centers are able to drive PUE down to below 1.1, most data centers are in the range of 1.6 to 2.5.
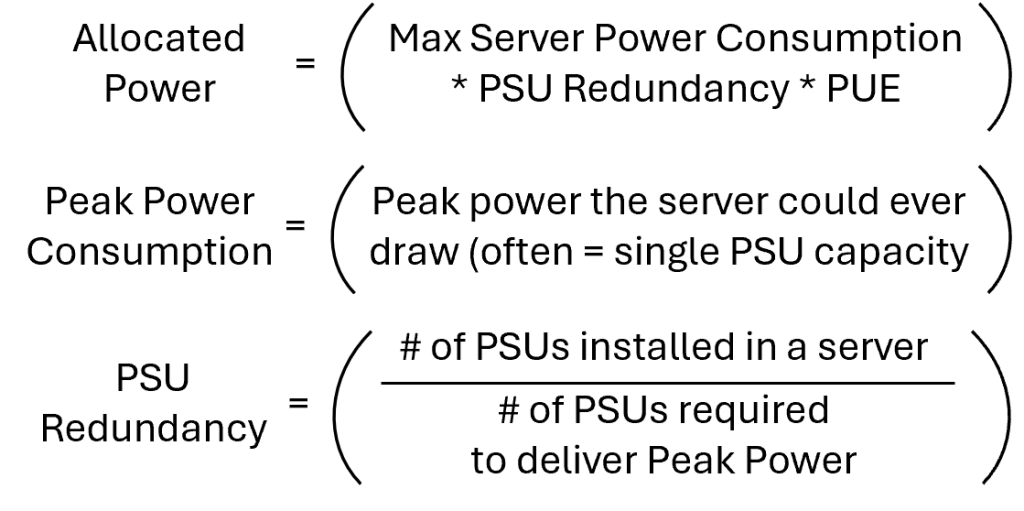
Using a typical 1600 watt server, and assuming a PUE of 2.0, the data center will need to size the power supply chain to support 6400 watts (1600 *2 for redundancy = 3200 * 2.0 to account for the power and cooling infrastructure = 6400 watts of allocated power). Remember that the supply chain includes the redundant PSUs, the redundant PDUs in the rack, the redundant PDUs at the end of the rack row, the entire cooling system, the backup power system and the utility feeds. This is of course justifiable if the IT equipment’s power consumption averages around 50%. However, if the utilization is actually below 25%, then quite a bit of the investment made in that supply chain has been wasted. The good news is that when that happens, there is extra capacity that could be reclaimed.
Impact of new computing paradigms
Today we are at the beginning of the AI and Machine Learning era and are dealing with the rapid expansion of new computing technology and infrastructure, and that is driving the massive need for more power (insert Star Trek reference here). This would be a bit concerning if we hadn’t seen it all before.
Conversations around power and cooling a couple of decades ago – during the mainframe era – were simple. Back then, you could toss another x86 server into the data center with little concern for cooling. There was enough cold air in the rooms that it didn’t really matter.
Then client-server computing created massive server farms, and data centers were rapidly heating up. The concept of having hot and cold aisles began to take shape, and the management of power and cooling became a big part of data center operations. Still, power consumption continued to grow at an unprecedented pace with single purpose “application” servers that were often overprovisioned, resulting in very low utilization rates averaging 10-15%. Data centers were over capacity with significant waste and inefficiency. This challenge drove an incredible level of innovation resulting, most importantly, in multiple virtualization technologies coming to the market.
Virtualization made a significant improvement, allowing data centers to take advantage of increasing server performance and collapsing multiple “single application” servers down to one more powerful virtualization server without requiring most of those applications to be rearchitected. This drove a very important innovation cycle that enabled data centers to regain control of server proliferation with a “virtualize first” approach. As virtualization technology matured and the open source options grew in capability, we began to see businesses offering hosted virtual servers. This evolution, combined with innovations in orchestration tools, enabled the beginning of Cloud Computing.
With Cloud Computing, processing could be completely outsourced, and Cloud providers could drive massive efficiency levels into their data centers – some were even cooling with outside air. They could use larger buildings, spread them out, optimize them for cooling in those environments, and locate them in places where power and cooling were more readily available.
But now we are in the era of AI and Machine Learning and organizations are making significant investments in accelerators to support their adoption efforts. Accelerators are purpose built and specialized, and each consume as much as 500 watts or more. It’s estimated that AI will contribute an additional 200 terawatt-hours per year to data center power consumption between 2023 and 2030.
What was a 1600-watt server with a couple of CPUs and memory is now a 10 kW server because it has multiple accelerators inside of it. The challenge is that according to the 2024 AFCOM State of the Data Center Report the average rack power is 12 KW. That means most data centers can only support one of these power hungry servers per rack. Given future accelerators are expected to exceed 1KW each, the industry is talking about 50, 100 and even 200 KW racks.
Data center operators and IT organizations are now challenged to provide capacity for these very expensive and important servers. Every 10 kW server actually requires 40 kW of power supply chain capacity. This challenge is compounded by the rapid pace of innovation in the accelerator technology to support this new era. With new accelerators are coming out every 9 to 18 months, IT organizations need to evaluate their impact on the power requirements of their data centers.
Calculating effective GPU usage
The rate of change and improvement in GPUs is staggering. What was deployed last year for your AI infrastructure is now being replaced or augmented with the next generation of technology that’s twice as capable, but also at least twice as power hungry. A 10 kW server is now approaching or exceeding 20 kW.
As we begin to add AI capacity into our data centers, we end up with a population of varied generations of accelerators where usage rates become unpredictable. The newest GPU servers will likely have high demand and operate optimally (since these were selected and designed for a specific purpose or application). However, last year’s GPU servers are, at best, in use 50% of the time, and that doesn’t factor in the application’s efficiency.
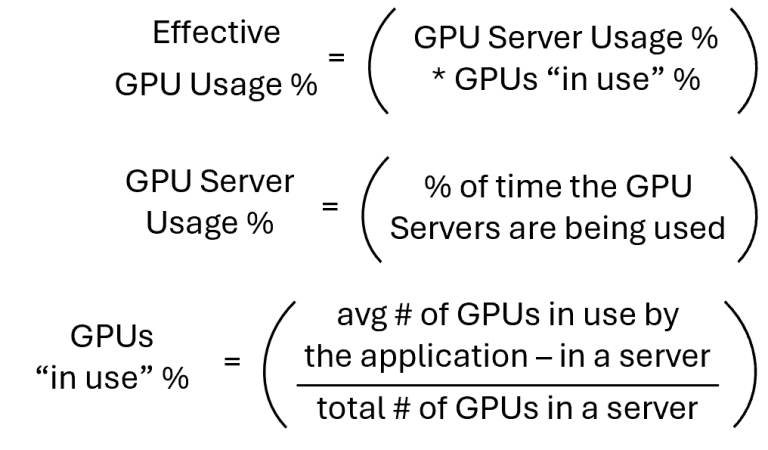
To understand this fully and to capture it in a way that allows us to appreciate the challenge, we will need to align on a calculation. We are not talking about utilization, which is driven primarily by the application and will vary by workload, rather we want to focus on how often the accelerators are “in use” (Effective GPU Usage %). There are two measurements (or estimates) needed: GPU Server Usage % – how often the GPU server is in use (running a job or workload) and when it is in use; and GPUs in Use”% – how many accelerators the application is using. The ability for an application to utilize all the accelerators is heavily dependent on the design of that application and its ability to “scale up” the number of accelerators. Often adding a second or third accelerator will not improve the performance and in some cases will slow down the application because of the communication overhead. If we have a GPU server that is “in use” 75% of the time, that GPU server has four (4) GPUs installed and the applications running on that GPU server use on average three (3) GPUs, then the Effective GPU Usage % = 75% * ( 3 / 4 ) = 50%.
An example data center scenario:
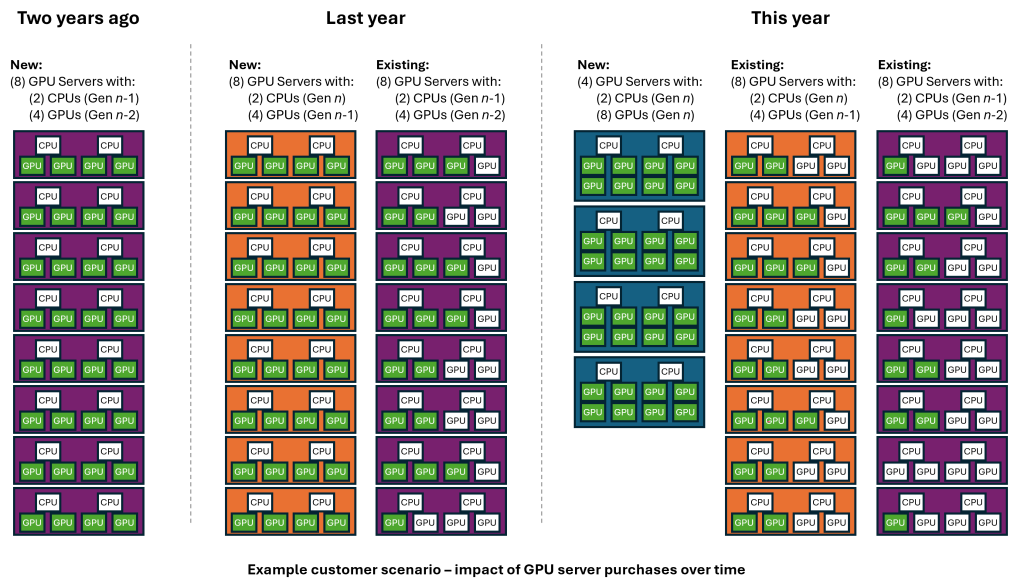
Leading-edge business applications, often new extensions of current applications, are driving the need for accelerated computing. These applications are at the “tip of the spear” with rapid optimizations to take advantage of the latest technology. Consider this example scenario that we often hear from data center customers:
Two years ago, IT purchased GPU servers to support a leading-edge application with four (4) GPUs per server of “n-2” GPU generation (where “n” = current generation).
A year ago, the leading-edge application required replacing those GPU servers with what we will now call “n-1” generation of GPU servers (again four GPUs per server). The older “n-2” servers were allocated to a second set of applications. However, although these applications benefited greatly, they did not take advantage of all four GPUs in each server – averaging ~2 to 3 GPUs in use. So, while the servers stayed busy, the GPUs in those servers were under-utilized.
This year, the leading-edge application again replaced those GPU servers with current “n” generation GPU servers with eight GPUs per server. The “n-1” GPU servers were allocated to the second set of applications which again benefited. However, with the newer technology the average number of GPUs in use actually fell to ~1 to 2 GPUs. Of course, the “n-2” GPU servers are now being used in a limited sense for new application testing, but the configuration (four per server) is suboptimal for proper application testing.
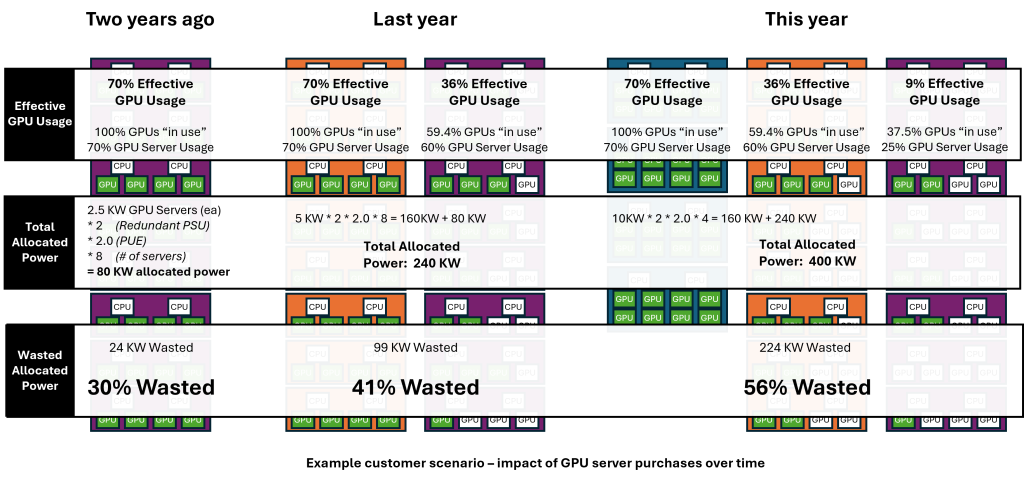
When we combine the impact of this typical purchase and usage pattern with the earlier discussion around power infrastructure and the necessary allocations for redundancy and utility requirements, we begin to see the size of the problem. In our basic example, 56% of the allocated power is wasted.
This scenario has played out in almost every data center with IT organizations working on creative ways to make the older generation of GPU servers (that still have significant value left) work for their needs. They are all looking for a better way…
Achieving efficiency in real-time
At Cerio, we help organizations take these new GPUs or accelerators into their environment and dynamically allocate them to the servers that are appropriate for each application with the ratios of GPUs to servers appropriate for those applications.
In the Cerio real-time system model, you can then take the prior generation GPUs and allocate them for other use cases. Like virtualization where you can dynamically ebb and flow your infrastructure, we can do that when allocating GPUs.
You can also take advantage of cutting-edge, purpose-built accelerators, test them with multiple applications, see their impact and compare that to prior results in a stepwise fashion appropriate for your environment. This provides a high level of control and optimized efficiency around usage but also lets you take advantage of the very latest technology at your pace, well ahead of when they may be available in a broader market, allowing you to deploy what’s best at the time you need it.
The traditional approach of deciding how many accelerators you will purchase based upon how many will fit in your approved vendor’s servers forces you to make compromises – are you purchasing more than you need? Less than you need? Do you need that new server? With a dynamic and composable solution, you can now allow the application to drive those decisions through actual usage and testing. When (not if) the algorithm changes or a new version of the application is released, it becomes very easy to compose the right GPUs or accelerators in the right quantity based upon the new requirements. Now you can buy servers when you need compute capacity and buy accelerators when you need accelerator capacity. This approach ensures that the investments you make in the accelerators and the entire power supply chain to support them is not wasted with under-subscribed GPU servers.
By enabling dynamic allocation of GPUs and accelerators, to the servers and applications that need them, IT organizations can drive maximum utilization of their entire fleet of these high value assets. This creates an infrastructure that allows you to be much finer grained with how you add future GPU and inference accelerators and will change the way you think about your AI infrastructure.
Shifting to sustainable systems
Data centers already consume 1-2% of the world’s electricity, and the challenges with power and cooling are significantly rising with AI adoption. Our customers estimate their effective GPU usage rate at well below 50%, with even lower rates for older generations.
Cerio enables you to reach maximum potential usage and utilization of accelerators in your data center so that they’re allocated where and when you need them and, more importantly, so that you don’t have to allocate power and cooling for idle resources. You don’t have to buy new GPUs until you need them, and you can purchase in smaller increments. With real-time systems, you’re composing your GPUs to the servers as needed, and can give them back to the pool for other servers to utilize when they’re not needed.
At Cerio, we grow with technology, with your applications, with your data center and your businesses so that your environment and power usage is fully optimized for effective usage and efficiency.