How GPU Density Needs Are Shaping Server Purchases
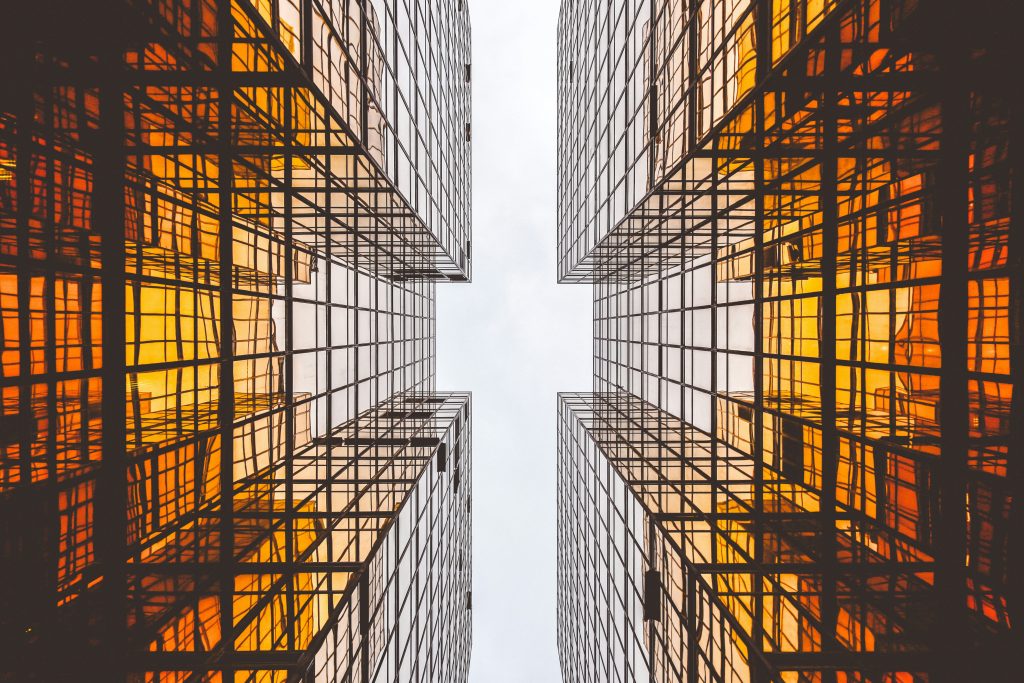
To support high-density GPUs for applications like AI and ML, the physical limits on the number of GPUs that can be attached to a server have driven the need for a new generation of servers. These servers must meet the escalating demands for power, cooling, and connectivity required to scale GPUs effectively. This presents challenges in cost, space, power, and cooling, forcing customers to frequently upgrade their server platforms as new generations of GPUs and accelerators are released. To overcome this heavy financial and operational burden, companies need the ability to:
- Use the servers they have in existing data centers without reinvesting in new server systems with every new accelerator advancement
- Optimize the balance of capacity and performance in their servers by adding accelerators and GPUs – without the drawback of relying on nonstandard server hardware
History
Decades ago, the shift from mainframes to industry-standard client-server machines led to design choices tailored to the applications of that era, ensuring the right balance of memory, storage, and compute capacity.
However, this model has remained largely unchanged for the past 30 years, built on the assumption that the CPU handles the majority of computational tasks, with most of the cost concentrated around it. As GPUs have become increasingly dominant for augmented processing, the traditional CPU-centric server design is no longer well-suited for today’s GPU-focused data centers.
Two socket system
At the physical level, industry standard servers have been optimized around a classic two CPU system that has sufficient power and cooling for components within that server.
Those converged platforms could include storage, large amounts of memory, as well as two CPU sockets. The two CPU sockets were primarily to give us access to even more memory, and as applications became more memory hungry, the amount of memory capacity and available memory bandwidth became a critical design consideration for server manufacturers.
Virtualization increased the demand for more CPU sockets, enabling the division of CPU resources across various applications. However, as workloads shift toward being more GPU-centric, this approach is no longer the most efficient or optimal for deployments that require greater access to GPUs.
Limitations of traditional server design
The problem is that the physical construction and design of standard platforms didn’t contemplate the shift toward more GPU-centric applications. The way that servers are managed and operated was still focused on a CPU centric design model. The number of physical devices that we might attach to that server was intentionally limited to simplify and focus development of servers for their initial purpose. But as we look at the scale of GPU deployments, some of those constraints are becoming inhibitors. Many server manufacturers have responded by building larger servers:
- Physically larger servers: Designed to hold more devices, these servers come with bigger power supplies, larger cooling fans, and occupy more rack space.
- Enhanced GPU support: Modifications such as updated system BIOS and operational adjustments have been implemented to support higher GPU densities more effectively.
This approach significantly increases server costs, particularly in terms of operational expenses for power, cooling, and system management.
For certain types of workloads, this makes absolute sense. Proprietary platforms have come into the market that are specifically designed only to support a very high density of GPUs. But for a large percentage of the data center market, they are too expensive, they occupy too much space, and they aren’t designed to support a variety of data center applications.
This is not to say that dedicated or proprietary systems do not have a place in higher end data centers or in situations where we need very specific design points for high density GPU servers.
For many data center operators, a more flexible approach is essential—one that enables the scaling of GPUs, as needed, across a wide range of servers. This flexibility allows GPUs to be reused in different parts of the data center, ensuring their efficient utilization across a broader range of applications.
To do this, we need to rethink not just how we build servers but how we deploy GPUs.
The industry has known, for some time, that the best solution to maximize the flexibility and utility of expensive compute and accelerator (GPU) resources in the data center is to disaggregate those elements from the server. By disaggregating GPUs, and other accelerators and compute elements from the server, oftentimes, lower-end servers can be used without requiring any changes to server design when accommodating either a different mix or a much larger capacity or variety of accelerators, such as GPUs.
Choose the GPU you need
Can I have the flexibility to run different vendor GPUs or accelerators in the same server running the same application? Can I have the flexibility to run the right GPU with the right server at the right time? What if I’m running a specific application that needs some alternate GPU vendors or alternative type of accelerator?
Disaggregation of the GPUs and accelerators means that the answer to these questions is yes, you can have the flexibility to run different vendor GPUs in the same server; yes, you can choose the right GPU for the job; yes, you can tee up a specific custom designed accelerator into that server without cracking open the lid of that server.
Disaggregation is vendor agnostic. There are different CPU vendor choices in the market that means we’re in a situation where if you’re a particular CPU consumer, you may be limited in which platforms you have depending on which systems in the market support the GPU and the CPU you require.
We need to break that limitation so there are better choices for CPUs, servers and GPUs. By giving customers more choice, we ultimately improve competitiveness and innovation in the market, and the cost of systems that can be built.
Supply chain issues
When we look at dedicated systems with dedicated design, we’re down to supply issues. Even though the market for high density servers is growing, the number of high density servers available is limited. Their cost is often prohibitively high. We want the choice to use whichever server for whichever GPU, ensuring availability, and the right scale and agility for use cases.
Another challenge is that the rate of innovation by the accelerator and GPU vendors is significantly faster than the delivery of new CPU technology. Typically, CPUs have a refresh rate of once every 18-24 months, whereas GPUs are typically refreshed every 12 months.
Decoupling the dependency of the server and CPU manufacturer from the GPU allows customers to take advantage of new technology when it suits them, as opposed to when there is an available refresh cycle – resulting in more choices for data center operators.
Conclusion
Given the growing demand for augmented compute with GPU technology, Cerio is excited to offer a new way to deploy GPUs in the data center. Cerio gives customers the flexibility to choose the ideal server – at the right scale, price, and availability – to support the GPU and density required.
Customers can reuse their existing investment in server and GPU technology but still take advantage of market innovation. Customers can move forward when it suits them, with incremental costs that are manageable within their infrastructure. This approach allows them to avoid being forced into decisions about GPU usage and server types, giving them greater flexibility in how they deploy and scale.
With Cerio’s CDI platform, any server can be transformed into a GPU server, configured with the exact GPU type and density enabling you to choose, in real-time, the optimal setup and scale for your deployments to match the demands of your applications.